
Is general artificial intelligence around the corner? Is it 3 to 8 years away? What if I told you it was? (What if I told you it wasn't?)
Marvin Minsky, who co-founded the MIT AI laboratory, has done work on cognition and AI. He is considered to be the father of AI. He once said that "... from three to eight years, we will have a machine with the general intelligence of an average human being. I mean a machine that will be able to read Shakespeare, grease a car, play office politics, tell a joke, have a fight."
When was this said? 1970.
There has always been hype around robots and robot intelligence, artificially created by man. When nothing came about from the big promises and hopes of a future, this field of research, AGI or AI, was abandoned by many thinkers for decades. Thus came the "AI Winter" where we have been slowly unthawing our way out of.
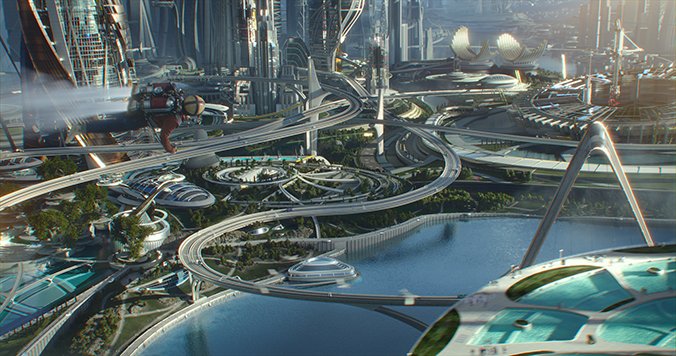
The dream of a techno robotic future was crushed. It's taken time to work up to that image and dream again. There is buzz anew surrounding AI and the deep learning being applied to beat humans in games like chess and Go.
The first early AI blunder was focusing on rule-based learning to try to emulate human reasoning. The lab results fuels the hype, and then when a report showed that all they were good at was in the lab band not rel world complexity, they funding dried up and so began the AI winter. No more government funding, no more students who instead sought out more promising careers.
But recently things have been moving to make AI more popular again. 1997's Deep Blue chess defeat of Kasparov. In 2005 131 miles was driven by an autonomous car. And in 2011 IBM's Watson beat two Jeopardy contestants. Having hit the mainstream again, AI was back in the hot seat and out of the cold long winter.
The savior was deep learning which forms a neural network hierarchy to filter information and make sense of the world. In looking at an object, its scans layers of various types, like layers of edges, texture, brightness, and so on. Then an object can be recognized from previous training. With enough training, patterns are developed and new unfamiliar objects can also be recognized as something as well.
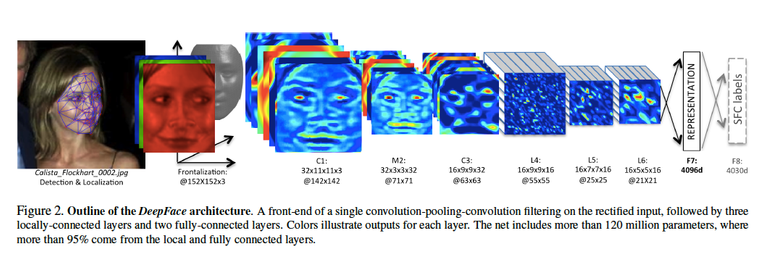
Much of the terminology we use towards AI is metaphorical and anthropomorphic. "Neural" network, "cognitive" computing, deep "learning". Machines don't think or learn. These are just familiar terms for us to simplify communication through referencing something to ourselves. This has been done for thousands of years as far as Egypt.
Even when we say AI, its not true AI yet. It's just computations being done, but not a free thinking artificial intelligence. Machine learning is not real learning. Neural networks are not neurons. Just to be clear. We should not mistake the metaphors for a literal reality. Some people don't make this distinction, and take the terms literally and put too much trust into the technology. When accidents can happen, such as with self-driving cars, then "AI" gets blamed, and the bubble pops again to another AI winter.
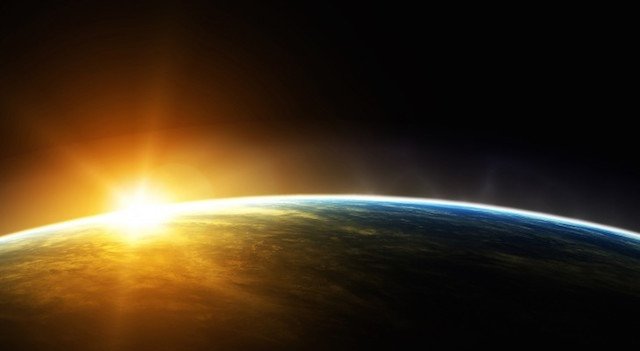
As was mentioned earlier on training, video is also used, not just pictures. A cat in a video can be recognized even though the "AI" system is not being trained for cats.
Many people think that with enough power, the programs could begin to learn on their own and go from their. So now there is an AI seduction going around. People don't understand how AI's function due to their level of complexity, so they imagine an emergent intelligence is on its way.
Rule-based mathematics, or deep learning, might never yield a real artificial intelligence. A cat is a cat, real or in a picture, for the "AI" we have now. The AI doesn't distinguish between the two. It doesn't have a real understanding of the world like a human.
This doesn't stop more students from taking up AI studies though. There is a new vision for AI in the future, and people are trying to get in early. Whether it pans out or not, has yet to be seen. The field of AI may be settings itself up for yet another fall if it focuses to heavily on one field again, like it did with rule-based learning. Deep learning may seem great now, but the limitations may show up sooner or later.
Here is the Gartner Hype Cycle. It shoots up with the hype, then falls, then levels off.
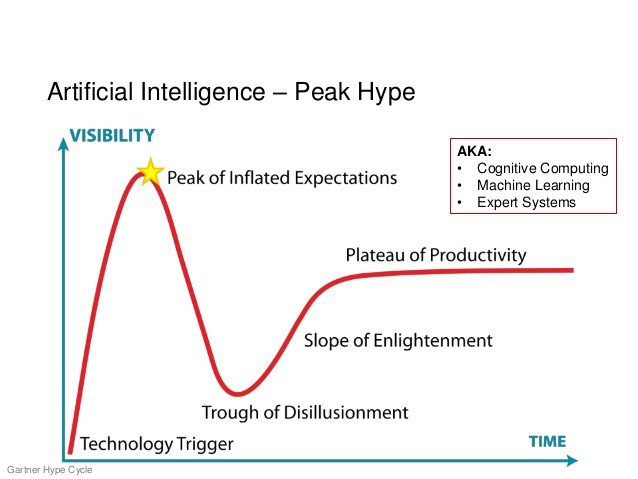
The super hyper around AI may lead to it's downfall again with more unrealistic expectation of it in the immediate future. But the mainstream keep chugging along the image of AI and the potential, with no signs of a bubble popping or that there is a bubble. AI is a hot topic with a lot of expected growth to come.
Here is a more detailed Gartner roadmap:

Maybe things will boom and more progress will be made, or maybe we will reach another block for a few decades because of over-hype of going in one direction again. For now, it's a hot investment that many people are trying to get into.
If you appreciate and value the content, please consider:
Upvoting , Sharing
and Reblogging
below.
Author: Kris Nelson / @krnel
Contact: [email protected]
Date: 2016-11-14, 7:12am EST
Hey Kris. Great post - upvoted and followed!
I knew they were mapping my face and all - good to know it's not a guy somewhere doing it. I am really sensitive about privacy (which is why I chose a sponge cartoon as my front). Anyway, keep posting! You will pop up on my feed now. Thanks
@sponge-bob I actually posted something about privacy: The scary “TRUTH” about social media FACE recognition software... @krnel interesting post...upvoted
Yeah, I saw that and upvoted it as well. Thanks @mokluc
Koo thanks ;) The mapping pic was just to show the layers they scan to identify faces. But yeah... intelligencia can do that using any camera out there... like in the Bourne movies LOL
The diffusion of the technology into libraries and frameworks is also important. I remember in the eighties it was all about abstract data types. Now days pretty much all you need is arrays, lists, and dictionaries. Wouldn't you say we should estimate how long it takes for a technology to be new to being just part of programmers toolkit?
That's another factor indeed. But hype cycle is more for adoption into the public domain of society, more than behind the scenes who is working on it, as I see it...
Still, cloud computing is in the 'trough of disillusionment', which is interesting since AWS, Azure, to some extent GCP, and also companies like Rackspace are already making quite a bit of money out of IaaS and PaaS.
Moreover, services like Netflix (AWS), Spotify (soon-ish GCP), Dropbox, iCloud, back-up providers, and many others have already made 'the cloud' accessible to the public without the public being aware of it.
Hi @krnel, an interesting post, and as always, a good read.
Thanks
I liked the reading of the part concerning machine learning, neural nets, etc... and AI. One easily call many things 'AI' that are just (sometimes complex) running algorithms. Which is a totally different thing from an AI.
Hey, that curve is exactly the same as the one for post ICO cryptos! Haha
Interesting piece. :)
It is in my opinion misleading to reduce deep learning (DL) to rule-based mathematics. Sure, software can be reduced to complicated if-then-else statements, but that misses the point: a deep learning network is a layered artificial neural network, which in itself evaluates functions. It is of course a mathematical model and it is implemented by software, but it distracts from the fact that a DL network is a very sophisticated model. After all, mathematicians have shown that a finite linear combinations of sigmoidal functions can approximate any continuous function of real variables (with support in the unit hypercube). A less technical discussion of so-called universality theorems can be found here.
The main difference between a simple rule-based model and one backed by neural networks (but also simpler machine learning algorithms) is that in a rule-based model you have to program all the branches, whereas in ML algorithms the algorithm determines the weights and relative importance of these weights, which in turn lead to 'branches', if you will. Someone still has to write an implementation of these algorithms, but by and large you don't consider, say, an optimization algorithm to be a collection of if-then-else statements. In the same way, few people think about programs as on/off of transistors.
At the moment, DL is mostly empirical. What is really lacking is a deep understanding of the inner workings. There seem to be a few ideas from physics that have parallels in deep learning (e.g. spin glasses and the renormalization group), but what these research articles do not show is the actual why. It's interesting that there may be a connection between deep learning and theoretical physics, but that does not really prove anything, just that the mathematics used to describe both is similar or even the same. Moreover, a lot of these theoretical comparisons use simplified networks (e.g. RBMs) and not the ones you would actually see in, say, Google DeepMind.
I said "or", this, or that, or the other, may not even yield the result we hope. It may lead to further developments to new technology that may though... I wasn't trying to equate them exactly. Thanks for the feedback.