Contributor
On a recent work trip, I found myself in a swanky-but-still-hip office of a private tech firm. I was drinking a freshly frothed cappuccino, eyeing a mini-fridge stocked with local beer, and standing amidst a group of hoodie-clad software developers typing away diligently at their laptops against a backdrop of Star Wars and xkcd comic wallpaper.
I wasn’t in Silicon Valley: I was in Johannesburg, South Africa, meeting with a firm that is designing machine learning (ML) tools for a local project backed by the U.S. Agency for International Development.
Around the world, tech startups are partnering with NGOs to bring machine learning and artificial intelligence (AI) to bear on problems that the international aid sector has wrestled with for decades. ML is uncovering new ways to increase crop yields for rural farmers. Computer vision lets us leverage aerial imagery to improve crisis relief efforts. Natural language processing helps usgauge community sentiment in poorly connected areas. I’m excited about what might come from all of this. I’m also worried.
AI and ML have huge promise, but they also have limitations. By nature, they learn from and mimic the status quo–whether or not that status quo is fair or just. We’ve seen AI or ML’s potential to hard-wire or amplify discrimination, exclude minorities, or just be rolled out without appropriate safeguards–so we know we should approach these tools with caution. Otherwise, we risk these technologies harming local communities, instead of being engines of progress.
Seemingly benign technical design choices can have far-reaching consequences. In model development, tradeoffs are everywhere. Some are obvious and easily quantifiable — like choosing to optimize a model for speed vs. precision. Sometimes it’s less clear. How you segment data or choose an output variable, for example, may affect predictive fairness across different sub-populations. You could end up tuning a model to excel for the majority while failing for a minority group.
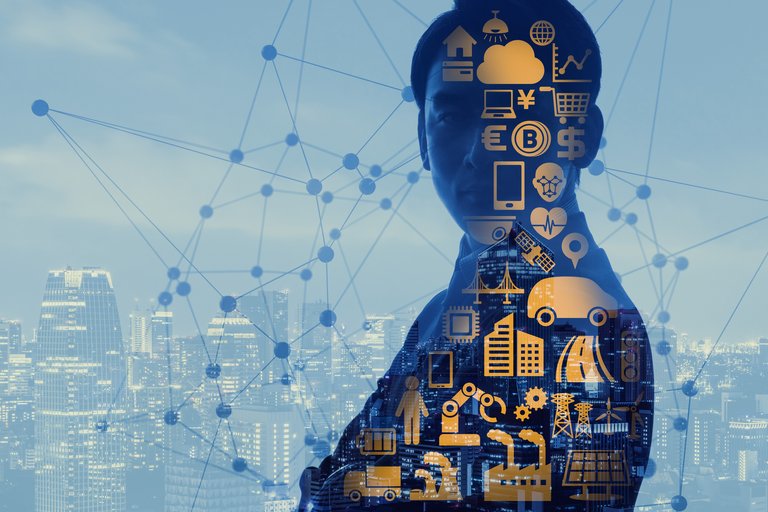
Image courtesy of Getty Images
These issues matter whether you’re working in Silicon Valley or South Africa, but they’re exacerbated in low-income countries. There is often limited local AI expertise to tap into, and the tools’ more troubling aspects can be compounded by histories of ethnic conflict or systemic exclusion. Based on ongoing research and interviews with aid workers and technology firms, we’ve learned five basic things to keep in mind when applying AI and ML in low-income countries:
- Ask who’s not at the table. Often, the people who build the technology are culturally or geographically removed from their customers. This can lead to user-experience failures like Alexa misunderstanding a person’s accent. Or worse. Distant designers may be ill-equipped to spot problems with fairness or representation. A good rule of thumb: if everyone involved in your project has a lot in common with you, then you should probably work hard to bring in new, local voices.
- Let other people check your work. Not everyone defines fairness the same way, and even really smart people have blind spots. If you share your training data, design to enable external auditing, or plan for online testing, you’ll help advance the field by providing an example of how to do things right. You’ll also share risk more broadly and better manage your own ignorance. In the end, you’ll probably end up building something that works better.
- Doubt your data. A lot of AI conversations assume that we’re swimming in data. In places like the U.S., this might be true. In other countries, it isn’t even close. As of 2017, less than a third of Africa’s 1.25 billion people were online. If you want to use online behavior to learn about Africans’ political views or tastes in cinema, your sample will be disproportionately urban, male, and wealthy. Generalize from there and you’re likely to run into trouble.
- Respect context. A model developed for a particular application may fail catastrophically when taken out of its original context. So pay attention to how things change in different use cases or regions. That may just mean retraining a classifier to recognize new types of buildings, or it could mean challenging ingrained assumptions about human behavior.
- Automate with care. Keeping humans ‘in the loop’ can slow things down, but their mental models are more nuanced and flexible than your algorithm. Especially when deploying in an unfamiliar environment, it’s safer to take baby steps and make sure things are working the way you thought they would. A poorly-vetted tool can do real harm to real people.
AI and ML are still finding their footing in emerging markets. We have the chance to thoughtfully construct how we build these tools into our work so that fairness, transparency, and a recognition of our own ignorance are part of our process from day one. Otherwise, we may ultimately alienate or harm people who are already at the margins.
The developers I met in South Africa have embraced these concepts. Their work with the non-profit Harambee Youth Employment Accelerator has been structured to balance the perspectives of both the coders and those with deep local expertise in youth unemployment; the software developers are even foregoing time at their hip offices to code alongside Harambee’s team. They’ve prioritized inclusivity and context, and they’re approaching the tools with healthy, methodical skepticism. Harambee clearly recognizes the potential of machine learning to help address youth unemployment in South Africa–and they also recognize how critical it is to ‘get it right’. Here’s hoping that trend catches on with other global startups too.
Powered by WPeMatico
This user is on the @buildawhale blacklist for one or more of the following reasons: