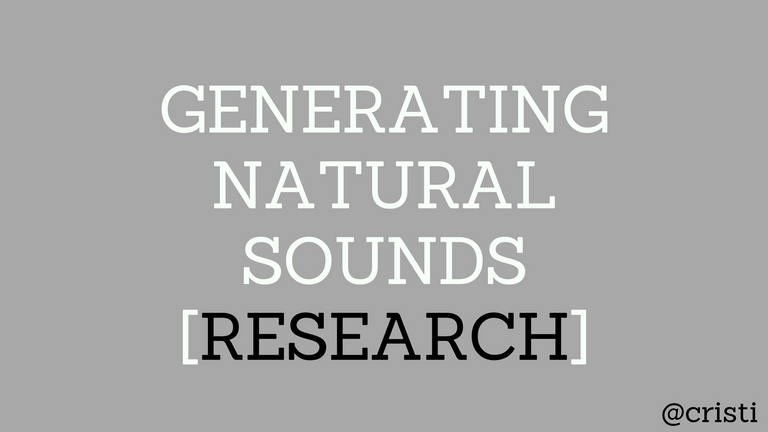
Zhou and colleagues (2017) recently published a paper in which they show how to generate sound from visual inputs. They start from the argument that human senses like sound and vision, during natural events, combine to provide a cohesive understanding of the world.
They think that applications of their work:
"...could help enable applications in virtual reality (generating sound for virtual scenes automatically) or provide additional accessibility to images or videos for people with visual impairments." [source]
In their paper they describe related work that has been conducted in this field, such as video and sound self-supervision, speech synthesis, and mapping visual to sound.
In the model, they use VEGAS, or the Visually Engaged and Grounded AudioSet:
" Its ontology includes events such as fowl, baby crying, engine sounds. Audioset consists of 10-second video clips (with audios) from Youtube. The presence of sounds has been manually verified." [source]
They discuss and provide the details for three tested methods:
- Frame-to-Frame Method
- Sequence-to-Sequence Method
- Flow-based Method
Then they go on and explain how the training process goes, from sound generation to and through the three methods and then they discuss about the numerical evaluation of their model (as well as a human evaluation of it). Interestingly:
"Evaluations show that over 70% of the generated sound from our models can fool humans into thinking that they are real." [source]
This paper is technical and may be unapproachable by the non-expert public. However, practitioners and researchers in the field might be interested in the methods, given the last citation I provided. Paper:
To stay in touch with me, follow @cristi
Cristi Vlad Self-Experimenter and Author
Thanks for sharing @cristi, nice your post about programmer... regaerd from me
Awesome post!!! Very interesting!!