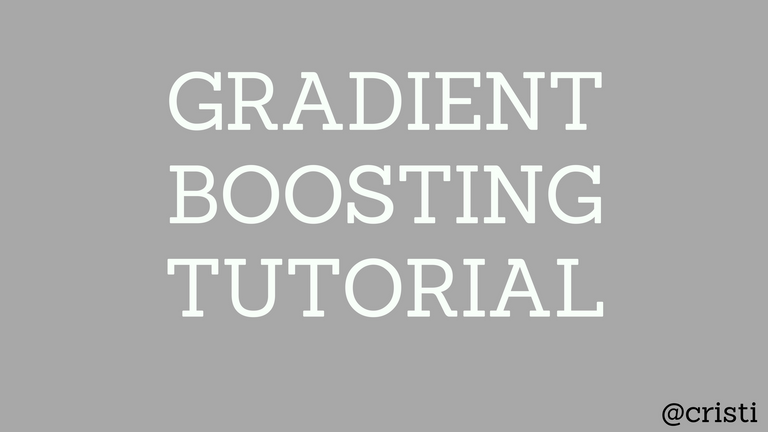
An algorithm that's worth looking into is Gradient Boosting. Alongside Random Forests, GBM is part of the ensembles category of ML algorithms.
In the past we've learned that Random Forests are comprised of Decision Trees (would you have even guessed?) and they augment the performance of single DTs quite significantly. Similarly, there are Gradient Boosting Regression Trees (GBRT) that are superior to DTs in most cases.
Today we'll stop specifically at Gradient Boosting and I'll recommend a detailed tutorial by Prince Grover, who is a Data Science Intern at Manifold.ai. His motivation to create the tutorial was that in many competitions, winner algorithms are stacks or ensembles of various models:
"The purpose of this post is to simplify a supposedly complex algorithm and to help the reader to understand the algorithm intuitively. I am going to explain the pure vanilla version of the gradient boosting algorithm and will share links for its different variants at the end." [source]
He goes over:
- ensembles, bagging and boosting (ensemble techniques)
- the details of GBM, the math and the intuition behind it
- how to fit a GBM
- how to visualize GMBs
He uses a code of Decision Trees from fast.ai on top of which he adds his own simple GBM model. For those who want to learn more, Grover shares and recommends a few resources for GBMs, including his own Github repo.
The full code for the tutorial is not in the post even though this was supposed to be a 'from scratch' tutorial. However, those are in the field might fill in the blanks with ease, while newbies, in my opinion, should start learning about simpler algorithms, like Logistic Regression and Decision Trees first, before getting into this type of stuff.
The tutorial is available below:
To stay in touch with me, follow @cristi
Cristi Vlad Self-Experimenter and Author
thank you @ full post @cristi, thanks for sharing