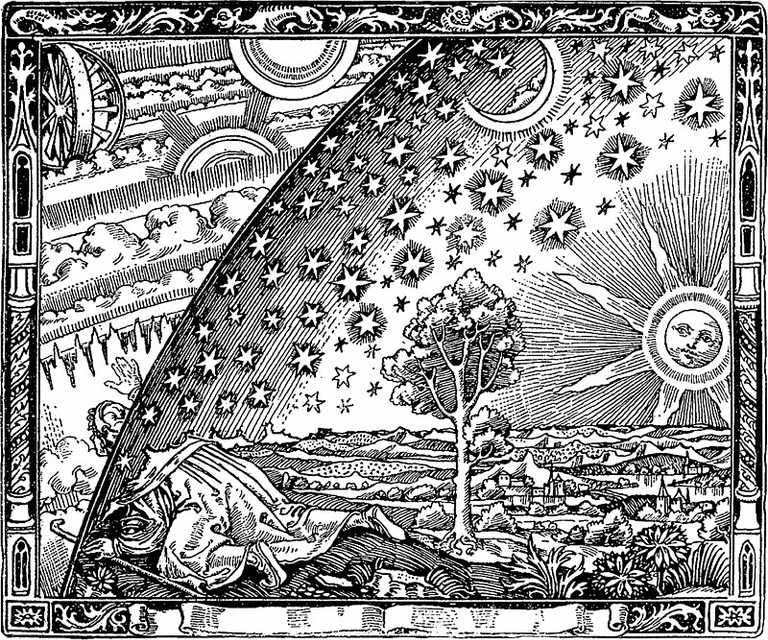
Imagine flipping a coin a thousand times, observing 506 heads and 496 tails. You conclude the coin is fair. How do you know for sure? Maybe the coin is in fact really unfair, but we happened to get lucky 506 times? Maybe there are some hidden variables through which a complex deterministic pattern of heads and tails arises. Are we doomed to never truly understand reality, being limited by our observations? What if there is no underlying reality and existence is just a string of meaningless experiences, audaciously leading us to believe in what amounts to nothing more than silly myths? Is there no escape from Plato's cave? Is life meaningless? These questions lead some people to adopt a religion and others to learn about bayesian inference.
Sorry I haven’t posted in a while. When I decided I wanted to write about Bayesian Inference I wasn’t sure if I wanted to use a mathematical or a more philosophical approach. In the end, I figured that the philosophical side has a whole dedicated community in forums like lesswrong and the mathematical side has been beaten to death by people who understand it a lot better than I do. This article is kind of a middle ground that touches a little of both.
Bayesian inference is, at its heart, a tool for finding the truth. Everything we know comes from making observations about the world. From these observations we try to hypothesize the underlying truth.
So what does knowing the truth even mean? From a practical perspective, knowing the truth allows you to predict the future. The more your understanding of the world reflects reality the more accurately you can predict future observations. Using the coin example again, if we are right about our assumption that the coin is fair we are able to predict future tosses.
Let’s say you set up a hypothesis about the underlying truth of a phenomenon. For it to be accepted as true it must predict future observations reasonably well. How do we know how well it predicts the future when we live in the present? We could wait for data from future observations to arrive, sure, but what if it is too late then? What good is predicting an increase in global warming, if we can only confirm it to be true when the polar ice caps have already melted? We need a way to be confident in our hypotheses now, not later.
The answer, of course, is through experimenting and collecting a lot of data. Naively, we might believe that, if our hypothesis explains this data well enough then surely it must be true. This doesn’t have to be the case.
While being good at predicting the future implies at least some utility in explaining the past the opposite is not true. We may have a model that explains the past perfectly but is horrible at predicting the future.
Let’s say we flip a coin and get heads, tails, tails, heads. We hypothesize, “whenever I flip this coin four times in a row I will get heads, tails, tails, heads in that order.” This hypothesis explains the past perfectly but it is horrible at predicting the future. We know this intuitively, because of our prior knowledge about how coins work.
That was a very basic example, but hopefully you believe me that we need prior knowledge about how well a hypothesis generalizes to future data to rate how good a hypothesis is.
This is actually quite a profound fact, as it makes us question whether it is possible to be completely unbiased and learn the truth from observations alone without preconceived notions about the world.
If we eliminate this prior knowledge when forming the hypothesis, we can still make sure the hypothesis explains the past data well, but we can’t make sure our knowledge will generalize to new observations. That is to say, we need prior knowledge to prevent ‘overfitting’.
We now have two methods of rating a hypothesis: how good it is at explaining data (people call this the “likelihood”) and how well it generalizes based on our prior knowledge (people call this the “prior”). If we multiply these two ratings we get a new rating called the posterior. This multiplication is the central idea in Bayes Theorem.

When does a hypothesis have a high posterior? If it is likely to generalize well (has a high prior) and it “fits” to our data (has a high likelihood) then the hypothesis has a high posterior rating as well.
On the other hand if our hypothesis has a low prior it needs to compensate by achieving a huge likelihood in order to compete with other hypotheses that have a high prior. You have definitely heard this said as “extraordinary claims require extraordinary evidence”.
If we have multiple hypotheses and we must decide which one more likely reflects the truth, we choose the one with the highest posterior rating (the “maximum a posteriori”). This is Bayesian inference.
Typically the hypotheses are statistical models and the prior, likelihood and posterior are actually expressed as probabilities, but the idea is exactly the same.
In summary, when there’s no time to test our hypotheses we need to find the truth based on what we already know. Bayesian inference lets us do this based on both the observations we have made and an intuitive feel for how probable a hypothesis is a priori.
Observations are like translucent windows on the house of truth: we need our intuition to know what is really going on inside.
Here is some more information on the prior.
Here is a great explanation of Bayes theorem.
Here is a great post on lesswrong about some of the implications Bayesianism has on rationality.
Here is a great explanation of statistical models in general.
It is always good to post the text like this because the probability, correlation and causality are the terms often not understood by the general public. I wrote about the article about the math and the anecdotes about the vaccines. Your other posts are also nice. https://steemit.com/health/@alexs1320/mathematics-of-vaccination
Thank you! I do my best. ^^