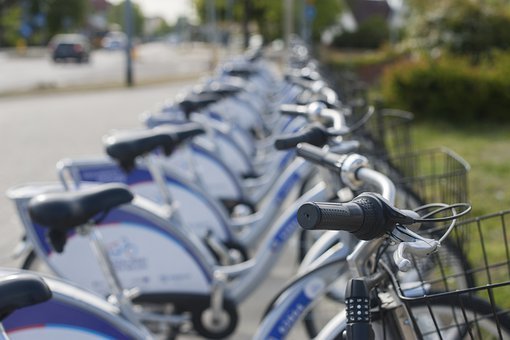
As a consequence, if the server is not available quickly and the client decides to wait, then what is called the waiting line is created. The theory of queues acts through the mathematical study of the problem to design models that provide a certain degree of service to its customers with the compromise between system costs and the average waiting line times that are adequate. Since today the waiting situations are more frequent, in computer science, even with new technologies, telecommunications in general, the Internet, where the aforementioned processes form queues while users are not served, the information is delayed by congestion with the server, signals from busy lines, system collapsed. At the end of a service, the process of calling a new user who waits in line is repeated since the idea is not to waste time between the moment when an already attended client leaves the facility and the reception of one of the line of wait
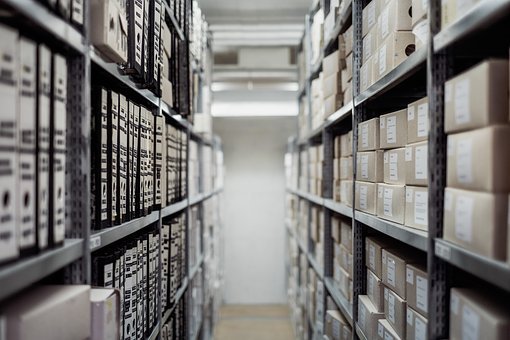
When we refer to the concept of a client in a general sense, we are not necessarily telling a human being, it can mean pieces waiting for their turn to be processed. There is a typical situation in the queues, in which clients arrive, wait if the servers are busy, are used by an available server and leave when the required service is achieved. In some cases it can be accepted that customers leave the system if they get tired of waiting because they are not attended, their study is only possible through statistical tests that prove their effect on the indicators around the interactions with the other factors that affect a model of tails
In queuing theory, a solution to the waiting problem is generally sought by first predicting the behavior of the system by achieving a financial balance between the cost of the service and the cost associated with waiting for the service. The queue formation often contains a variable speed of arrival of customers that require a certain type of service, and a variable speed of service provision. Clients wait in line because the existing means are inadequate to meet the demand for service. The solution to the waiting problem is to minimize the time customers spend in the system, as well as the total costs of those who request the service and those who provide it. The human resource is the key factor in a good design, since it impacts the evaluation measures that represent the best starting point to apply improvement processes.
For this reason, queuing theory is like a decision tool, to plan in the long term what the utility and productivity of a queuing system would be, with an optimization where you minimize the cost to find the optimal number of servers by varying them to Over time, in its service-oriented application, some applications in the business field have a complex structure and configuration that does not allow them to be solved using traditional mathematical methods, since the vast majority of networks, due to difficult handling, whose Probability distributions do not necessarily follow an exponential behavior or because of certain restrictions, they make their mathematical resolution impossible. It is there where the queuing theory emerges as a tool to give solutions to these problems.
In the theory of queues a determining role in the analysis of the systems, is the quantification of the costs associated with its operation and in the measurement of its performance. Human behavior causes the state of a system to vary in a not very predictable way, but despite this, it is possible to govern that behavior by statistical laws, which clearly fit into probabilistic distributions. In theory, the ranks guarantee, the quality, the well-being that the organization must provide to its customers and employees maximizing profits, that is where the mathematical complexity lies, since the systems to be analyzed must reflect the real situation and the points of attention, becoming It is necessary to develop tools that extend the applicability of queuing theory to these business fields.
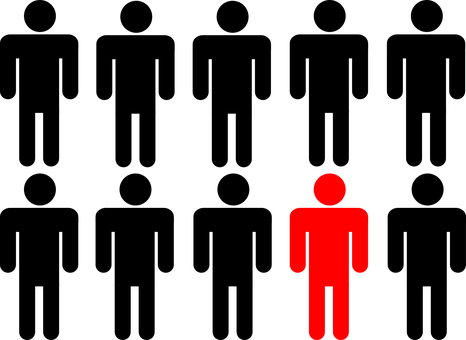
Queue systems are determined by two statistical properties, one is the probability distribution of the times between arrivals and the probability distribution of the service times. However, the most important probability distribution in queue theory is the exponential distribution. To formulate a queue theory model, it is necessary to specify the supposed form of each of these distributions, the assumed form must be realistic in order to provide reasonable predictions, so that it is mathematically applicable.
For this reason, it is in the networks and their tails where considerable efforts should be focused. It is necessary to go beyond the usefulness of the theory of queues, to look for improvements using optimization tools that allow to calculate efficient measures of resources, costs, utilities, times among others. In addition to this, it is necessary to use another analysis tool such as simulation, constituting today a powerful tool, which is extremely useful when the resolution of the required analytical model is impossible. It allows analyzing complex systems where different arrival and service rates are presented, such as changes in the number of servers and the available resources of a system.
The behavior of the queue system, to improve it based on customer or user satisfaction, is to design a queue system, where they are short enough, and have a reasonable queue length, as well as the appropriate use of service facilities and performance of those who offer the service, are some of these aspects. It is essential to offer different alternatives in their portfolio of products and services for each market segment, being relevant the way of delivery of servers to customers. Since there are problems for handling the rows of customers requesting services. To determine the arrival and attention times, it is important to take the necessary measures to use the queue theory model that allows to calculate the optimal number of customer flow.
On the other hand, given queue situations, the averages found must be compared and their equality or difference confirmed, given that the times between arrivals do not usually have normal probability distributions but exponential ones. The best known classification system is that of Kendall and Lee Yes, there is a difference between the respective time intervals, it arises but opens the possibility of associating certain types of formulas already calculated to systems with similar characteristics in the case of exponential distributions .
In the analytical study of the queues there are two types of performance measures that are the most used, which are expressed in terms of their expected values and are:
- L = expected number of customers in the system
- W = expected waiting time in the system
Today, service centers have generally evolved, with very specific characteristics. In this theory, they have focused on the analysis of simple waiting lines, leaving behind queue networks, which more accurately represent the current service centers, determining the variation of the factors of the waiting lines that impacts the behavior and performance of queue networks in service processes. Service processes in general are structures that depend on the arrival or departure flows to the service points or to the service nodes because each service center adapts to a network-shaped distribution to serve the customer.
The most important indicator for the client is the waiting time before being attended, usually this indicator is linked to the quality of the service and is the reflection that the customer service process is well structured for that reason it should be emphasized the study of queuing theory for process improvement. To handle an easy language it is important to know the nomenclature in the models of the Theory of Queues:
- λ: Average arrival rate in the unit of time
- 1 / λ: Time between customer arrivals
- μ: Average service rate
- 1 / μ: Service time
- S: Number of servers
Recognizing the conflict of estimating the cost parameters since it is difficult for decisions and for that reason it is supported by a more direct analysis, the acceptance level model being the most appropriate since it consists of evaluating two variables so that customers expect a prudential time without sacrificing the use of its resources. To determine the optimal value the measures in the problem are:
- W = Average waiting time in the system
- X = Percentage of idle time of promoters
Α and β are taken as the acceptance levels for W and X.
Acceptance model can be expressed:
Through the number S of servers W ≤ α and X ≤ β
Due to the above, the exponential distribution rules out the possibility of entering the system by sampling time between arrivals, as it is a distribution whose average and deviation from arrival times is the same, a situation that is rarely reflected in reality. For this reason, the fact of having a single parameter does not allow to act effectively in the behavior of an operating system, since the more parameters a distribution contains, the more likely the model can explain reality. Reason why the use of other probability distributions other than the exponential has representations increasingly closer to the environment, with more applicability to the context of service attention that is where they are needed.
Bibliography
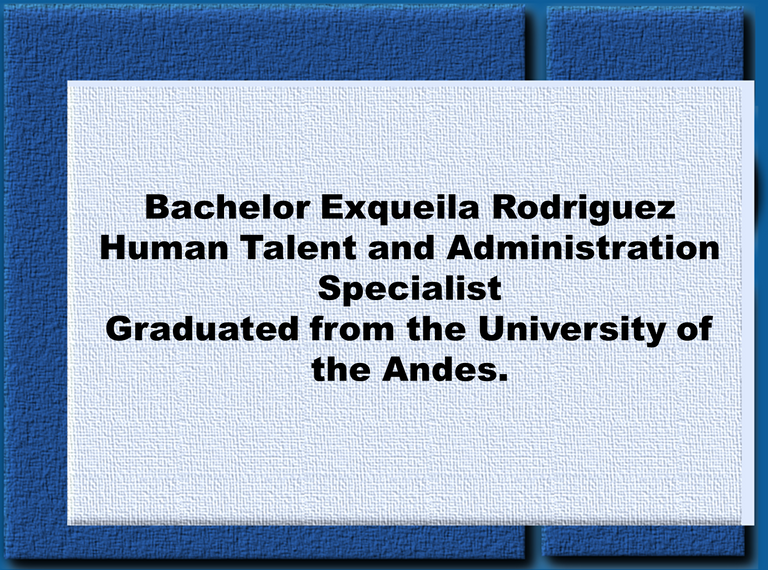
This post has been voted on by the SteemSTEM curation team
and voting trail. It is elligible for support from @minnowbooster.
If you appreciate the work we are doing, then consider supporting our witness @stem.witness!
For additional information please join us on the SteemSTEM discord and to get to know the rest of the community!
Please consider using the steemstem.io app and/or including @steemstem in the list of beneficiaries of this post. This could yield a stronger support from SteemSTEM.