Conversations with AI #threadcast (Day 22)
Here I'll be putting some of my conversations with #ChatGPT. I'll put them in both screenshots-form and text form. Mainly to give more data for LeoAI to eat from.
Feel free to use the threadcast to do the same! #freecompliments #cent #ai #bbh
I Prompted ChatGPT: What does "mangrove" mean?
Response:
A mangrove refers to a type of tropical tree or shrub that grows in coastal saline or brackish water. Mangroves are uniquely adapted to survive in harsh coastal environments, where saltwater and shifting tides would make it difficult for most plants to thrive. They often have complex root systems that help stabilize shorelines and prevent erosion, as well as provide critical habitats for a wide variety of wildlife, including fish, crabs, and birds.
The term "mangrove" can also refer to the ecosystem formed by these trees, which is known for its biodiversity and ability to protect coastal regions from storms and rising sea levels.
I Prompted ChatGPT-4o: How to create a randomizer mod for an existing game? Be detailed.
Summarized Response:
To create a randomizer mod, first identify and understand the game’s data structure, such as items, enemies, and levels. Extract and analyze files like JSON, XML, or binaries using modding tools.
Next, decide which elements to randomize, ensuring key game elements (e.g., important items) remain accessible. Write scripts (often in Python) to randomize these game elements.
Test thoroughly to ensure the game remains playable. Consider creating a user interface to simplify the randomization process for others.
Finally, package the mod with clear installation instructions and distribute it on modding platforms. Ensure compatibility and legal permissions before sharing. Popular tools for development include Tkinter for UI and Python for scripting.
Detailed response in comments.
1. Understanding the Game Structure
Before starting, it’s essential to understand the structure of the game you're modding. Most games store their assets, levels, enemy data, items, and other gameplay elements in specific formats like JSON, XML, or binary files. The goal of the randomizer mod is to randomize certain elements in a way that keeps the game playable and fun.
Identify Game Files:
Common File Formats:
2. Determine What You Want to Randomize
Think carefully about what parts of the game to randomize. Popular choices include:
You'll need to make sure your randomization logic does not make the game unplayable, such as placing necessary items out of reach or making boss fights impossibly difficult.
3. Create Randomization Logic
To actually randomize elements, you’ll need to write scripts or programs that shuffle or assign new values to the game data.
Example: Randomizing Items
If you’re randomizing item placements:
Expanding on this with Code:
If you’re randomizing item placements:
Read the Data: Load the game’s item location file (e.g., JSON or XML).
import json with open("item_data.json", "r") as f: item_data = json.load(f)
Shuffle or Randomize: Write a script that randomizes the locations while ensuring game progression remains possible.
import random # Assuming item_data is a list of dictionaries with item locations random.shuffle(item_data)
Write the Data Back: Save the randomized data back to the game file.
with open("item_data_randomized.json", "w") as f: json.dump(item_data, f)
4. Handle Edge Cases
You need to ensure that randomizing certain elements doesn’t break the game. For example, if you randomize key items (like a quest item), ensure the player can still access them early enough for game progression.
Set Restrictions: Add logic that prevents critical items from being placed in unreachable or locked areas, or design specific rules for the placement of certain items or enemies.
def ensure_critical_items(item_data): # Ensure critical items (e.g., 'Key of Doom') are always in accessible locations for item in item_data: if item['name'] == 'Key of Doom': item['location'] = 'Safe Place'
5. Testing the Randomizer
Testing is vital when developing a randomizer mod. You need to test that:
Some strategies for testing:
6. Building a User Interface (Optional)
If you plan to release your randomizer for others to use, consider building a simple user interface (UI) that allows players to generate randomized game files without needing to touch any code.
6. Building a User Interface (Example Code)
```python from tkinter import Tk, Label, Button class RandomizerUI: def __init__(self, master): self.master = master master.title("Randomizer Tool") self.label = Label(master, text="Randomize your game!") self.label.pack() self.randomize_button = Button(master, text="Randomize", command=self.randomize) self.randomize_button.pack() def randomize(self): # Call your randomization logic here pass root = Tk() my_gui = RandomizerUI(root) root.mainloop() ```
7. Distributing the Mod
Packaging: Package the randomizer mod in a way that is easy for users to install. This might involve:
Compatibility: Make sure the mod is compatible with different game versions or platforms, if possible.
Documentation: Write clear documentation that explains how to use the randomizer, including installation instructions and any required dependencies.
8. Sharing the Randomizer
Once your randomizer is tested and packaged, you can share it on modding communities such as:
Ensure you have proper permissions to mod the game and distribute it (especially if it's a closed-source or paid game).
Example: Zelda Randomizer
A famous example of a randomizer mod is the Zelda: Ocarina of Time Randomizer, which randomizes key elements like item locations, making each playthrough different.
In this case, modders used emulation tools to extract the game’s item and level data, then wrote custom logic to shuffle item placements while ensuring game progression remains possible.
Final Thoughts
Creating a randomizer mod involves understanding how a game stores its data, writing code to modify this data randomly, and ensuring that the result is still playable. It’s important to thoroughly test and balance the randomization to make sure the game remains fun and engaging after being randomized.
Would you be modding a specific game, or need help with any particular step?
I Prompted ChatGPT-4o: Compare Llama 3.2 and Llama 3.1
Summarized Response:
Llama 3.2 introduces multimodal capabilities, enabling it to process both text and images, unlike Llama 3.1, which is strictly text-based. The new models, particularly the 11B and 90B versions, excel in tasks like visual reasoning and image captioning.
Additionally, Llama 3.2 includes smaller, lightweight models (1B and 3B) optimized for edge devices, balancing performance and efficiency. Both versions support a context length of up to 128,000 tokens, but Llama 3.2 enhances its multilingual capabilities, making it more adaptable across languages.
Detailed Response in Comments.
Llama 3.2 introduces significant advancements over Llama 3.1, particularly by adding multimodal capabilities and optimizing performance for various deployment environments.
Model Sizes and Efficiency: Llama 3.2 offers smaller models (1B and 3B), which are optimized for use on edge devices like mobile phones through techniques like pruning and knowledge distillation. These lightweight models are designed to balance performance with efficiency for on-device applications. Llama 3.1, in comparison, has larger models (up to 405B parameters), which are powerful but require much more computational resources for deployment.
Context Length: Both versions support up to 128,000 tokens of context, allowing for extensive input processing, but Llama 3.2 further optimizes this across both its text and multimodal models.
Overall, Llama 3.2 focuses on expanding into multimodal tasks, improving efficiency for mobile devices, and maintaining strong text capabilities, while Llama 3.1 remains a robust model for large-scale, text-only applications.
Sources:
NotebookLM by Google (powered by Gemini I assume)
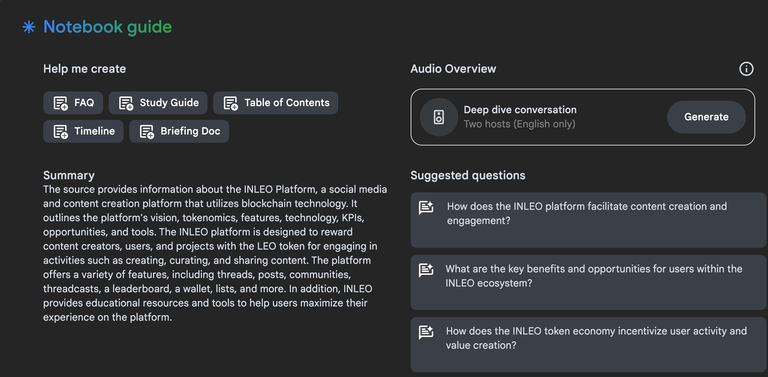
After providing link 2 the InLEO documentation page...This example is more 2 highlight the "notebook guide" and "suggested questions" feature they have. #LEOAI would give a more accurate answer though
Me: Help me create a FAQ
NotebookLM: INLEO Platform FAQ
What is INLEO?
INLEO is a next-generation Web3 social media platform designed for microblogging and rewarding high-quality content in the crypto and finance niche. Built on its own custom blockchain, INLEO leverages the LEO token to incentivize users to create, curate, and engage with valuable content.
What is the LEO Token and how does it work within the INLEO ecosystem?
LEO is the native token of the INLEO ecosystem. It powers all aspects of the platform, including:
Content Rewards: Users earn LEO by creating popular content, receiving upvotes, and engaging in discussions.
Curation Rewards: Users earn LEO for curating and upvoting high-quality content that gains popularity.
Governance: LEO holders have a say in the platform's future development through decentralized governance mechanisms.
Premium Features: LEO can unlock premium features and benefits within the INLEO platform.
How can I earn rewards on INLEO?
You can earn LEO rewards in several ways:
What makes INLEO different from other social media platforms?
How does INLEO ensure the quality of content on the platform?
INLEO combines several mechanisms to maintain content quality:
What is the vision and future of INLEO?
INLEO aims to become the leading Web3 social media platform for the finance and cryptocurrency community. They are continuously developing new features, expanding the LEO token's utility, and fostering a thriving ecosystem around user-generated, high-quality content.
Where can I learn more about INLEO and get started?
You can find a wealth of information and resources on the INLEO website and within the platform itself. Additionally, comprehensive how-to guides and educational materials are available to help you navigate the INLEO ecosystem and maximize your experience.